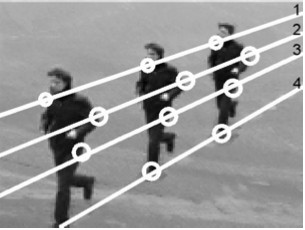
Segmentation and Reconstruction from Periodic Motion
Ongoing Project
Project Lead(s)
Synopsis
A method for detecting and segmenting periodic motion is presented. We exploit periodicity as a cue and detect periodic motion in complex scenes where common methods for motion segmentation are likely to fail. We note that periodic motion detection can be seen as an approximate case of sequence alignment where an image sequence is matched to itself over one or more periods of time. To use this observation, we first consider alignment of two video sequences obtained by independently moving cameras. Under assumption of constant translation, the fundamental matrices and the homographies are shown to be time-linear matrix functions. These dynamic quantities can be estimated by matching corresponding space-time points with similar local motion and shape. For periodic motion, we match corresponding points across periods and develop a RANSAC procedure to simultaneously estimate the period and the dynamic geometric transformations between periodic views. Using this method, we demonstrate detection and segmentation of human periodic motion in complex scenes with non-rigid backgrounds, moving camera and motion parallax.
Project Details
Non UCSD Vision People also Involved
Ivan Laptev and Patrick Pérez (IRISA/INRIA)